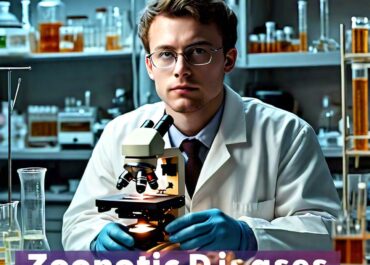
Differences Between AI in Business Process Automation and AI in Data Analytics
AI in business process automation and AI in data analytics represent two critical applications of artificial intelligence (AI) in modern business operations. AI in business process automation (BPA) focuses on automating repetitive, time-consuming tasks, freeing up employees to focus on higher-value activities. It involves using AI-driven systems like robotic process automation (RPA), machine learning, and natural language processing (NLP) to optimize workflows and streamline operations. This type of automation leads to enhanced efficiency, cost savings, and reduced human error across various industries, such as finance, manufacturing, healthcare, and customer service.
On the other hand, AI in data analytics refers to the application of machine learning and other AI technologies to process, analyze, and derive insights from large datasets. With vast amounts of data being generated every day, businesses leverage AI to identify patterns, trends, and correlations that would otherwise go unnoticed. By applying AI-driven analytics, companies can make data-driven decisions, predict future trends, and gain a competitive advantage. Both AI in business process automation and data analytics play pivotal roles in modern organizations, but they differ significantly in terms of focus, application, and outcomes.
AI in Business Process Automation Overview
AI in business process automation (BPA) is the application of AI technologies to automate repetitive, rule-based tasks and processes, improving efficiency and reducing the need for manual labor. Below are five key aspects of AI in business process automation.
1. Automation of Repetitive Tasks
AI in BPA is designed to automate mundane and repetitive tasks that consume time and resources. Processes such as data entry, invoice processing, and customer service inquiries are prime candidates for automation. AI-powered systems like robotic process automation (RPA) can mimic human actions in interacting with digital systems, completing tasks faster and with fewer errors.
- RPA: Software robots that automate repetitive tasks such as copying data between systems.
- Time Savings: Automating these tasks allows employees to focus on more strategic and creative work.
2. Enhancing Workflow Efficiency
AI in BPA improves workflow efficiency by streamlining operations across departments. AI can help manage workflows by identifying bottlenecks, optimizing task allocation, and ensuring that resources are used efficiently. By continuously learning from patterns in business processes, AI systems can make recommendations for process improvements.
- Workflow Optimization: AI identifies inefficiencies in workflows and suggests improvements.
- Resource Allocation: AI helps allocate resources more effectively based on workload and priorities.
3. Reducing Operational Costs
One of the major advantages of AI in BPA is the reduction in operational costs. By automating repetitive tasks, businesses can significantly reduce the costs associated with manual labor and human error. AI-powered systems work 24/7 without fatigue, ensuring higher productivity and accuracy, which leads to long-term cost savings.
- Cost Savings: Automation reduces the need for human intervention, lowering labor costs.
- Error Reduction: Fewer human errors lead to less rework and reduced operational waste.
4. Improving Customer Experience
AI-driven automation also enhances customer experience by providing faster and more accurate responses. Chatbots and virtual assistants are commonly used in customer service to handle inquiries, resolve issues, and guide customers through processes. These AI tools can operate around the clock, improving customer satisfaction by offering instant support and reducing wait times.
- Chatbots and Virtual Assistants: AI systems that handle customer inquiries in real-time, improving response times.
- 24/7 Availability: Automation allows businesses to provide continuous customer support.
5. Scalability and Flexibility
AI-powered business process automation is highly scalable, allowing businesses to handle increased workloads without the need for additional resources. As businesses grow, AI systems can scale with them, automating more tasks and handling larger amounts of data. Additionally, these systems can be customized to fit specific industry needs, offering flexibility in how they are deployed.
- Scalability: AI systems can easily scale to handle growing workloads.
- Customization: AI automation solutions can be tailored to the specific needs of an industry or business.
AI in Data Analytics Overview
AI in data analytics refers to the use of machine learning algorithms and other AI technologies to analyze large datasets and extract meaningful insights. This enables businesses to make data-driven decisions and improve their overall performance. Below are five key aspects of AI in data analytics.
1. Processing Large Datasets
AI in data analytics excels at processing large volumes of data quickly and efficiently. Traditional data analytics methods may struggle to handle the sheer amount of data generated in modern businesses, but AI can process vast datasets in real time, identifying trends and patterns that would otherwise go unnoticed.
- Big Data Processing: AI processes and analyzes massive datasets with speed and precision.
- Real-Time Analysis: AI can provide insights in real-time, allowing businesses to respond quickly to changing conditions.
2. Predictive Analytics
A major advantage of AI in data analytics is the ability to perform predictive analytics. By analyzing historical data, AI algorithms can predict future trends, helping businesses make proactive decisions. Predictive models can forecast sales, customer behavior, market trends, and more, enabling companies to stay ahead of their competition.
- Predictive Models: AI uses past data to forecast future trends and outcomes.
- Business Forecasting: Predictive analytics helps businesses anticipate customer needs and market changes.
3. Improving Decision-Making
AI-driven data analytics enables businesses to make smarter, data-driven decisions. By uncovering hidden patterns, correlations, and insights from data, AI helps executives and managers make more informed decisions, minimizing the risks associated with intuition-based decision-making.
- Data-Driven Decisions: AI provides actionable insights that guide strategic decision-making.
- Reduced Risk: Making decisions based on data reduces the likelihood of errors and misjudgments.
4. Personalization and Customer Insights
AI in data analytics plays a significant role in improving personalization. By analyzing customer data, AI can identify preferences, behaviors, and purchasing patterns, allowing businesses to create personalized marketing strategies and enhance customer engagement. This is especially valuable in industries like e-commerce, where personalization leads to higher customer satisfaction and loyalty.
- Personalized Marketing: AI analyzes customer data to tailor marketing campaigns to individual preferences.
- Customer Insights: AI provides a deeper understanding of customer behaviors and preferences.
5. Anomaly Detection and Risk Management
AI in data analytics is highly effective at detecting anomalies in data, such as unusual patterns that may indicate fraud, system failures, or security breaches. This helps businesses manage risks more effectively by identifying potential issues before they escalate. In sectors like finance and cybersecurity, AI-powered anomaly detection is crucial for preventing fraud and maintaining system integrity.
- Fraud Detection: AI identifies unusual patterns in financial transactions that may indicate fraud.
- Risk Management: AI helps businesses mitigate risks by detecting anomalies and alerting stakeholders to potential issues.
Differences Between AI in Business Process Automation and AI in Data Analytics
- Focus
- AI in Business Process Automation: Focuses on automating repetitive tasks and workflows.
- AI in Data Analytics: Focuses on analyzing data to extract insights and inform decision-making.
- Primary Function
- AI in Business Process Automation: Automates operational processes.
- AI in Data Analytics: Analyzes and interprets large datasets.
- Goal
- AI in Business Process Automation: Increases efficiency and reduces human intervention.
- AI in Data Analytics: Helps make data-driven decisions and forecasts.
- Technology
- AI in Business Process Automation: Often uses RPA, NLP, and machine learning.
- AI in Data Analytics: Primarily relies on machine learning, deep learning, and data mining techniques.
- Scope of Application
- AI in Business Process Automation: Applied to operational tasks across various industries (e.g., customer service, HR).
- AI in Data Analytics: Applied to data processing and decision-making in areas like finance, marketing, and supply chain.
- Outcome
- AI in Business Process Automation: Frees up human resources and improves workflow efficiency.
- AI in Data Analytics: Provides actionable insights and predictions based on data.
- Real-Time Use
- AI in Business Process Automation: Executes tasks in real-time (e.g., processing orders, responding to emails).
- AI in Data Analytics: Analyzes real-time or historical data for insights and forecasts.
- Customization
- AI in Business Process Automation: Can be tailored to specific tasks within different industries.
- AI in Data Analytics: Customization is focused on the algorithms used to interpret specific datasets.
- User Interaction
- AI in Business Process Automation: Typically involves minimal user interaction after initial setup.
- AI in Data Analytics: Involves more user interaction for interpreting results and making decisions.
- Risk Management
- AI in Business Process Automation: Minimizes operational risks by reducing human error.
- AI in Data Analytics: Identifies risks through data patterns and anomaly detection.
Conclusion
Both AI in business process automation and AI in data analytics represent significant advances in how businesses operate and make decisions. AI in BPA enhances operational efficiency by automating repetitive tasks, reducing errors, and cutting costs, making it invaluable for improving day-to-day business functions. On the other hand, AI in data analytics allows businesses to leverage data-driven insights, enhancing their ability to make strategic decisions, predict future trends, and understand customer behavior.
While these two applications of AI serve different purposes, they both contribute to greater efficiency, improved decision-making, and a competitive edge in today’s fast-paced business environment. Understanding the differences between them allows businesses to harness AI effectively for both process optimization and data-driven innovation.
FAQs
Related Topics
- All
- Animals
- Diseases
- Health
- Money
- Politics
© 2024 OnYelp.com. All rights reserved. Terms and Conditions | Contact Us | About us